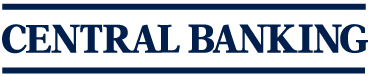
Techforward Award: Making Text Count – economic forecasting using newspaper text

The Covid-19 pandemic struck global economies with such force and speed that many central banks temporarily abandoned their headline forecasts in favour of scenario-based analysis. But new research published by the Bank of England (BoE) might help forecasters cope with such rapid movements as well as the underlying structural shifts in the economy.
In Making text count – Economic forecasting using newspaper text, Eleni Kalamara, Arthur Turrell, Chris Redl, George Kapetanios and Sujit Kapadia base their analysis on text drawn from three popular UK newspapers. They show text can improve forecasts of a wide range of macroeconomic variables – and the method works best during periods of stress.
By applying weightings to a large dictionary of common terms, a machine learning algorithm is able to focus on terms that are particularly important at any given time. The authors update the weightings for every 36 months of data, helping to illustrate how a central bank might use text data in its regular forecasting rounds.
The paper fills a gap in the literature, as machine learning and text mining have typically been applied more narrowly in the past, for instance to generate early-warning indicators of financial stress, rather than to forecast a wide range of variables.
A related innovation is the authors’ methodology. They generate a large number of regressors from the text and turn each into a time series for the machine learning algorithm to crunch through. Kalamara explains that running such huge datasets through a simple regression model could lead to overfitting, and therefore poor out-of-sample performance.
“We achieved higher predictive performance, especially during periods of stress, where, most of the time, more standard, traditional models fail to capture the swings in economic activity,” says Kalamara. “The neural net seems to capture the non-linearities that happen at that time.”
Kapadia notes the model is not only fast, but also seems to capture some additional information – it “can enhance forecasts at a horizon of up to nine months ahead”. One possibility is that newspaper text contains information that standard forecasts miss. Another is that newspapers may shape sentiment, he suggests.
Redl mentions a further benefit, in that the weightings on different terms mean a user can see what is driving a particular result. That is critical in presenting a convincing narrative to policy-makers.
Indeed, practical applications may not be far off. Turrell explains: “I have been working with Eleni to get some of these methods ‘productionised’, so forecast data is available to people at the BoE, should they wish to use them as an input – either visually or as a formal statistical input into other models. It is also worth stressing that, obviously, people use a wide range of models and model inputs – what we’re doing here is producing an extra input.”
Only users who have a paid subscription or are part of a corporate subscription are able to print or copy content.
To access these options, along with all other subscription benefits, please contact info@centralbanking.com or view our subscription options here: http://subscriptions.centralbanking.com/subscribe
You are currently unable to print this content. Please contact info@centralbanking.com to find out more.
You are currently unable to copy this content. Please contact info@centralbanking.com to find out more.
Copyright Infopro Digital Limited. All rights reserved.
As outlined in our terms and conditions, https://www.infopro-digital.com/terms-and-conditions/subscriptions/ (point 2.4), printing is limited to a single copy.
If you would like to purchase additional rights please email info@centralbanking.com
Copyright Infopro Digital Limited. All rights reserved.
You may share this content using our article tools. As outlined in our terms and conditions, https://www.infopro-digital.com/terms-and-conditions/subscriptions/ (clause 2.4), an Authorised User may only make one copy of the materials for their own personal use. You must also comply with the restrictions in clause 2.5.
If you would like to purchase additional rights please email info@centralbanking.com